Master the Art of A/B Testing with the Right Sample Size
Discover why sample size matters, how to calculate it, and when to apply it to ensure your tests lead to actionable insights.
Accurate Sample Sizes: Get precise calculations to avoid underpowered or overpowered tests.
Easy to Use: Input your data and get results in seconds—no complex math required!
Optimize Your Tests: Ensure reliable results with the right sample sizes.
Enhance Decision-Making: Make informed choices backed by solid data.
100% Free | No credit card required
No sign-up required
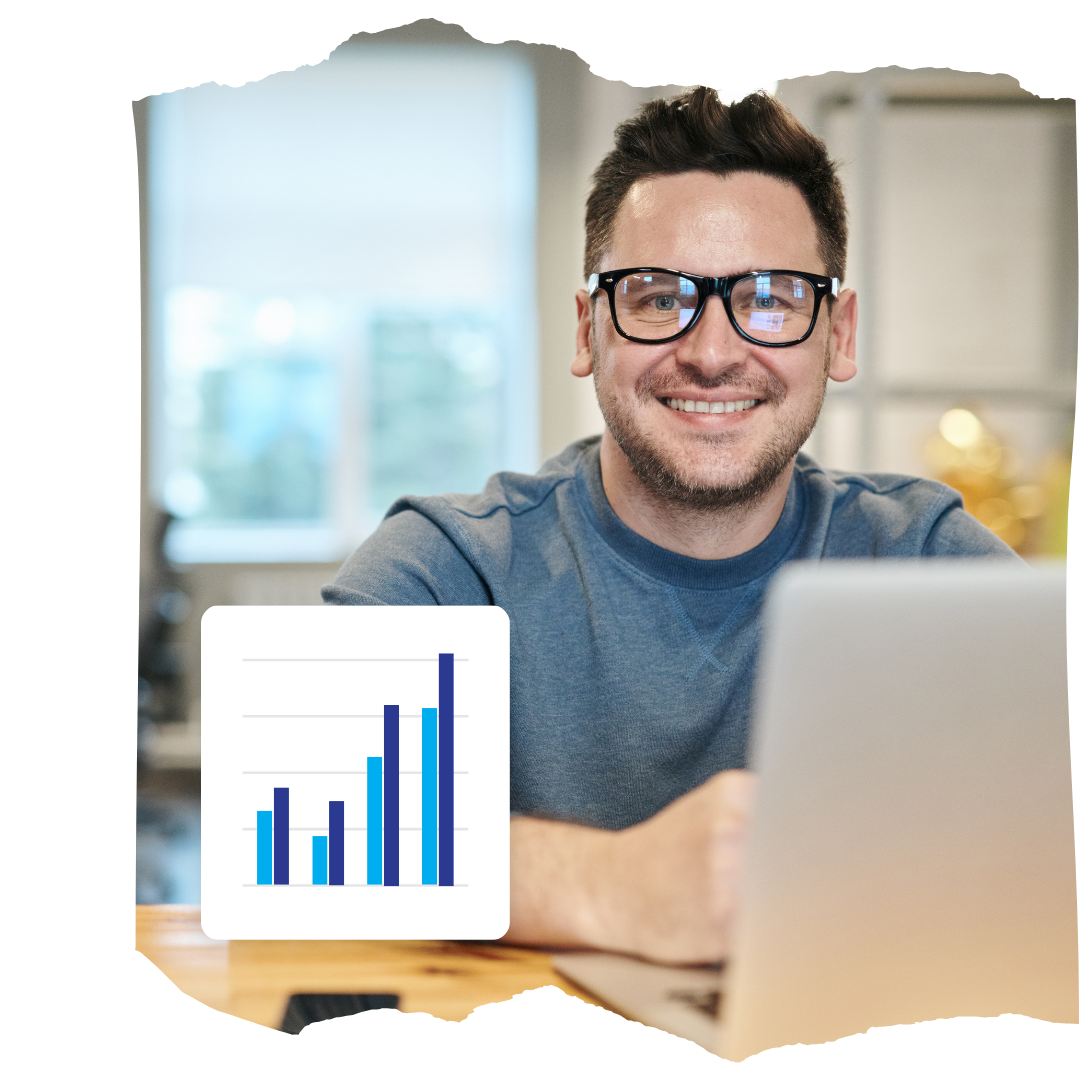
Sample Size is Crucial in A/B Testing
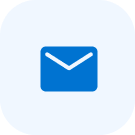
Statistical Significance
A larger sample size means you can be more confident that any observed differences between variations are not due to random chance. Smaller samples might lead to misleading results.
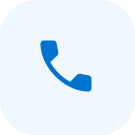
Accuracy of Results
With a larger sample size, reduces the margin of error and increases the reliability of your findings, helping you make better-informed decisions based on the data.
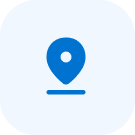
Ability to Detect Smaller Effects
A larger sample size increases the power of your test, allowing you to detect smaller differences between the variations.
I’m Jane Denzi, and I specialise In Optimising Digital Experiences through A/B Testing and rigorous Experimentation.
As a CRO expert, I understand that sample size is a crucial component in achieving accurate and actionable results. My passion lies in enhancing user engagement and conversion rates by meticulously analyzing data and refining strategies to deliver impactful outcomes.
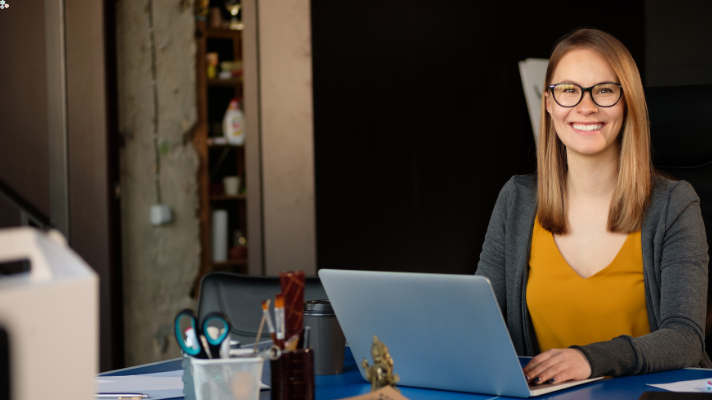
A/B Testing Sample Size Calculator
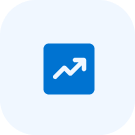
How To Use The Sample Size Calculator?
Purpose: Help users determine the ideal sample size needed for their A/B tests to ensure statistically valid results and reliable conclusions.
Key Components:
- Baseline Conversion Rate: Current conversion rate of the control group or existing variant.
- Minimum Detectable Effect (MDE): The smallest difference in conversion rates that the user wants to detect.
- Desired Confidence Level: The probability that the results are not due to random chance (e.g., 95% confidence level).
- Desired Statistical Power: The likelihood of detecting an effect if there is one (e.g., 80% power).
- Sample Size Output: Calculates the number of participants needed in each group (control and variant) to achieve the desired statistical power and confidence level.
User Flow:
- Input Parameters: Enter the desired confidence level, statistical power, minimum detectable effect, and baseline conversion rate.
- Calculate Sample Size: The tool computes the required sample size for each group based on the provided inputs.
- Review and Adjust: Review the suggested sample size and adjust inputs as needed to refine their test parameters.
Request New Features or Leave a Review
Optimize Your Testing with Perfect Sample Sizes
The sample size is rounded up to ensure the test maintains adequate statistical power and confidence.
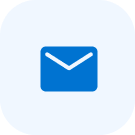
SPONSORED SECTION
Drop us an email via the contact us page for Pricing
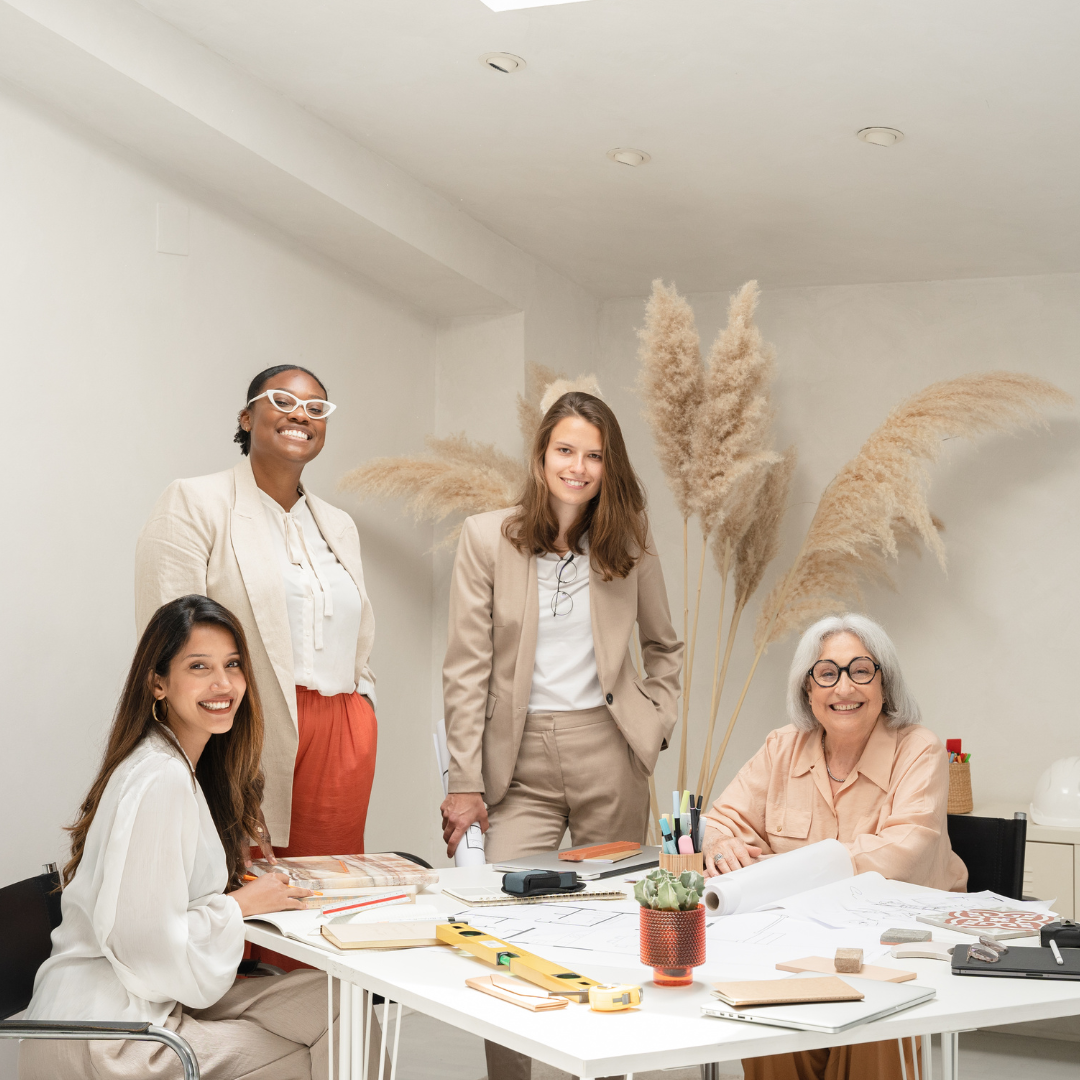
What Is Sample Size?
Sample size refers to the number of observations or participants used in a study or experiment. In Conversion Rate Optimisation (CRO), determining the correct sample size is essential to ensure that your test results are statistically significant and reliable. A sample that’s too small may lead to inconclusive results, while a sample that’s too large could waste resources.
Importance of Sample Size in CRO?
1. Statistical Significance
One of the most critical aspects of CRO is achieving statistical significance, which indicates that the results of your test are likely not due to chance. A well-calculated sample size ensures that the data collected will provide enough evidence to confirm whether a variation truly outperforms the control.
2. Confidence Levels:
The confidence level is the probability that the result of your test reflects the true nature of the population. Typically set at 95%, it’s directly influenced by your sample size. A larger sample size allows for a higher confidence level, which increases the reliability of your results.
3. Avoiding Type I and Type II Errors:
Type I Error (False Positive):
Concluding that a variation has an effect when it actually doesn’t.
Type II Error (False Negative):
Concluding that a variation doesn’t have an effect when it actually does.
A properly determined sample size helps minimize the risks of these errors, ensuring that your conclusions are accurate.
The Impact of Sample Size on Test Outcomes
1. Impact on Test Duration: Sample size directly impacts how long a test will need to run to achieve statistical significance. Larger sample sizes can lead to faster conclusions, but they also require more traffic or participants. Smaller sample sizes may take longer to produce conclusive results, risking external factors influencing the data.
2. Conversion Rate Fluctuations: Smaller sample sizes can lead to higher variability in conversion rates, making it difficult to discern real patterns from random noise. A larger sample size stabilizes conversion rate measurements, allowing you to detect even subtle changes in performance between variations.
3. Resource Allocation: Calculating the correct sample size ensures efficient use of resources. Running tests with either too few or too many participants can lead to wasted time, effort, and money. Proper sample size calculation allows you to optimize your resources and focus on tests with the highest potential impact.
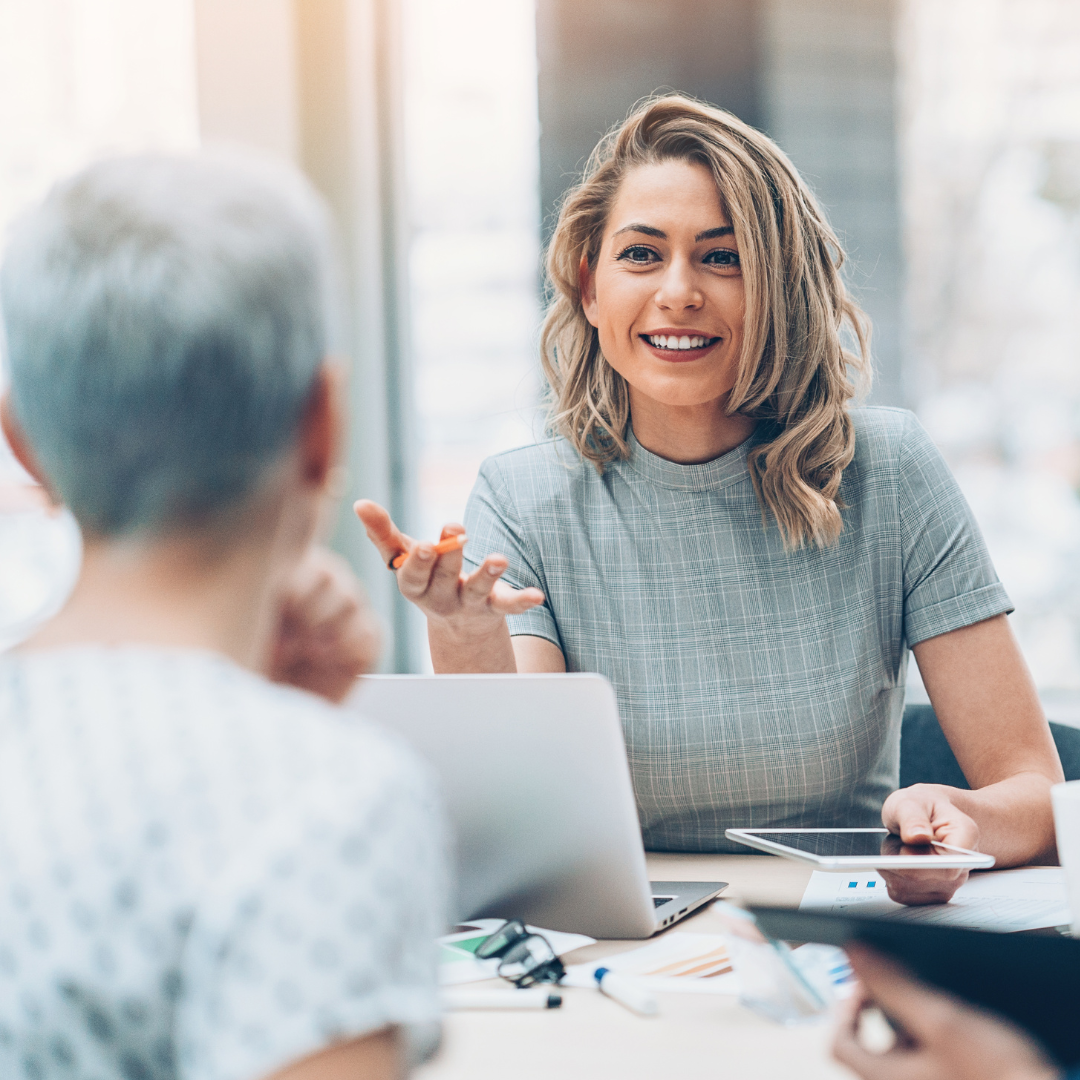
Key Factors Influencing Sample Size Calculation
1. Baseline Conversion Rate:
The existing conversion rate of your control page or element is a starting point for sample size calculation. The lower the baseline rate, the larger the sample size required to detect significant changes.
2. Minimum Detectable Effect (MDE):
MDE refers to the smallest change in conversion rate that you consider significant. If you’re aiming to detect small improvements, you’ll need a larger sample size to ensure those changes are statistically significant.
3. Confidence Level and Power:
Power: Often set at 80%, this represents the probability of correctly rejecting the null hypothesis when it’s false. Higher power levels require larger sample sizes.
Confidence Level: Typically set at 95%, this reflects how sure you are that the results are not due to random chance.
4. Traffic Volume:
The amount of traffic or participants available for testing influences the time it takes to reach the required sample size. High-traffic websites can achieve larger sample sizes quickly, enabling faster testing cycles.